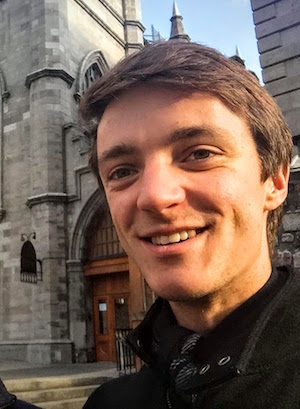
Hi, I’m Jon Gauthier. I’m a postdoctoral scholar working in the
Chang Lab at the University of California, San Francisco. I build
computational models of how people understand and produce language, and use
them to explain human behavior and brain activity, combining methods
from artificial intelligence, linguistics, neuroscience,
and philosophy.
I completed my Ph.D. in cognitive science at the MIT
Department of Brain and Cognitive Sciences in the Computational
Psycholinguistics Laboratory. You can find my full CV here.
I had the good fortune to begin research at a young age, thanks to the
generosity and support of my advisors and academic community. I’m interested
in helping ambitious undergraduate students likewise break into the world of
academia. Please get in touch!
Recent personal news
- I joined the Chang Lab in September 2023. Excited to develop new methods
and designs for studying the neural representation of linguistic structure!
- We’ve just launched LM Zoo, an open source repository of
state-of-the-art neural network language models.
- The Open Philanthropy Project has named me an AI Fellow.
We’ll be collaborating to support the development of more interpretable,
robust systems for language understanding, and the discussion of both short-
and long-term potential risks of artificial intelligence.
- I joined the MIT Department of Brain and Cognitive Sciences in
September 2017.
Research
(Find me on Google Scholar for an up-to-date list.)
The neural dynamics of auditory word recognition and integration.
Jon Gauthier and Roger Levy.
EMNLP 2023.
@inproceedings{gauthier-levy-2023-neural,
title = "The neural dynamics of word recognition and integration",
author = "Gauthier, Jon and
Levy, Roger",
editor = "Bouamor, Houda and
Pino, Juan and
Bali, Kalika",
booktitle = "Proceedings of the 2023 Conference on Empirical Methods in Natural Language Processing",
month = dec,
year = "2023",
address = "Singapore",
publisher = "Association for Computational Linguistics",
url = "https://aclanthology.org/2023.emnlp-main.62",
doi = "10.18653/v1/2023.emnlp-main.62",
pages = "980--995",
abstract = "Listeners recognize and integrate words in rapid and noisy everyday speech by combining expectations about upcoming content with incremental sensory evidence. We present a computational model of word recognition which formalizes this perceptual process in Bayesian decision theory. We fit this model to explain scalp EEG signals recorded as subjects passively listened to a fictional story, revealing both the dynamics of the online auditory word recognition process and the neural correlates of the recognition and integration of words. The model reveals distinct neural processing of words depending on whether or not they can be quickly recognized. While all words trigger a neural response characteristic of probabilistic integration {---} voltage modulations predicted by a word{'}s surprisal in context {---} these modulations are amplified for words which require more than roughly 150 ms of input to be recognized. We observe no difference in the latency of these neural responses according to words{'} recognition times. Our results support a two-part model of speech comprehension, combining an eager and rapid process of word recognition with a temporally independent process of word integration. However, we also developed alternative models of the scalp EEG signal not incorporating word recognition dynamics which showed similar performance improvements. We discuss potential future modeling steps which may help to separate these hypotheses.",
}
The neural dynamics of auditory word recognition and integration.
Jon Gauthier and Roger Levy.
CCN 2023.
@InProceedings{gauthier2023neural,
author = {Gauthier, Jon and Levy, Roger},
title = {The neural dynamics of auditory word recognition and integration},
booktitle = {Proceedings of the 7th Conference on Cognitive Computational Neuroscience},
month = {August},
year = {2023},
address = {Oxford, England}
}
Language model acceptability judgements are not always robust to context.
Koustuv Sinha, Jon Gauthier, Aaron Mueller, Kanishka Misra, Keren Fuentes, Roger Levy, and Adina Williams.
ACL 2023.
Outstanding Paper award (top ~2% of accepted papers)
@inproceedings{sinha-etal-2023-language,
title = "Language model acceptability judgements are not always robust to context",
author = "Sinha, Koustuv and
Gauthier, Jon and
Mueller, Aaron and
Misra, Kanishka and
Fuentes, Keren and
Levy, Roger and
Williams, Adina",
booktitle = "Proceedings of the 61st Annual Meeting of the Association for Computational Linguistics (Volume 1: Long Papers)",
month = jul,
year = "2023",
address = "Toronto, Canada",
publisher = "Association for Computational Linguistics",
url = "https://aclanthology.org/2023.acl-long.333",
pages = "6043--6063",
abstract = "Targeted syntactic evaluations of language models ask whether models show stable preferences for syntactically acceptable content over minimal-pair unacceptable inputs. Our best syntactic evaluation datasets, however, provide substantially less linguistic context than models receive during pretraining. This mismatch raises an important question: how robust are models{'} syntactic judgements across different contexts? In this paper, we vary the input contexts based on: length, the types of syntactic phenomena it contains, and whether or not there are grammatical violations. We find that model judgements are generally robust when placed in randomly sampled linguistic contexts, but are unstable when contexts match the test stimuli in syntactic structure. Among all tested models (GPT-2 and five variants of OPT), we find that model performance is affected when we provided contexts with matching syntactic structure: performance significantly improves when contexts are acceptable, and it significantly declines when they are unacceptable. This effect is amplified by the length of the context, except for unrelated inputs. We show that these changes in model performance are not explainable by acceptability-preserving syntactic perturbations. This sensitivity to highly specific syntactic features of the context can only be explained by the models{'} implicit in-context learning abilities.",
}
SyntaxGym: An online platform for targeted syntactic evaluation of language models.
Jon Gauthier, Jennifer Hu, Ethan Wilcox, Peng Qian, and Roger Levy.
ACL 2020 System Demonstrations.
@inproceedings{gauthier-etal-2020-syntaxgym,
title = "{S}yntax{G}ym: An Online Platform for Targeted Evaluation of Language Models",
author = "Gauthier, Jon and
Hu, Jennifer and
Wilcox, Ethan and
Qian, Peng and
Levy, Roger",
editor = "Celikyilmaz, Asli and
Wen, Tsung-Hsien",
booktitle = "Proceedings of the 58th Annual Meeting of the Association for Computational Linguistics: System Demonstrations",
month = jul,
year = "2020",
address = "Online",
publisher = "Association for Computational Linguistics",
url = "https://aclanthology.org/2020.acl-demos.10",
doi = "10.18653/v1/2020.acl-demos.10",
pages = "70--76",
abstract = "Targeted syntactic evaluations have yielded insights into the generalizations learned by neural network language models. However, this line of research requires an uncommon confluence of skills: both the theoretical knowledge needed to design controlled psycholinguistic experiments, and the technical proficiency needed to train and deploy large-scale language models. We present SyntaxGym, an online platform designed to make targeted evaluations accessible to both experts in NLP and linguistics, reproducible across computing environments, and standardized following the norms of psycholinguistic experimental design. This paper releases two tools of independent value for the computational linguistics community: 1. A website, syntaxgym.org, which centralizes the process of targeted syntactic evaluation and provides easy tools for analysis and visualization; 2. Two command-line tools, {`}syntaxgym{`} and {`}lm-zoo{`}, which allow any user to reproduce targeted syntactic evaluations and general language model inference on their own machine.",
}
A systematic assessment of syntactic generalization in neural language models.
Jennifer Hu, Jon Gauthier, Peng Qian, Ethan Wilcox, and Roger Levy.
ACL 2020.
@inproceedings{hu2020systematic,
author = {Hu, Jennifer and Gauthier, Jon and Qian, Peng and Wilcox, Ethan and Levy, Roger},
title = {A systematic assessment of syntactic generalization in neural language models},
booktitle = {Proceedings of the Association of Computational Linguistics},
year = {2020}
}
On the predictive power of neural language models for human real-time comprehension behavior.
Ethan Gotlieb Wilcox, Jon Gauthier, Jennifer Hu, Peng Qian, and Roger Levy.
ACL 2020.
@inproceedings{wilcox-etal:2020-on-the-predictive-power,
year = {2020},
title = {On the Predictive Power of Neural Language Models for Human
Real-Time Comprehension Behavior},
pages = {1707–1713},
booktitle = {Proceedings of the 42nd Annual Meeting of the Cognitive
Science Society},
author = {Wilcox, Ethan Gotlieb and Gauthier, Jon and Hu, Jennifer and
Qian, Peng and Levy, Roger P.}
}
From mental representations to neural codes: A multilevel approach.
Jon Gauthier, João Loula, Eli Pollock, Tyler Brooke Wilson, and Catherine Wong.
Behavioral and Brain Sciences.
@article{gauthier2019from,
title={From mental representations to neural codes: A multilevel approach},
volume={42},
DOI={10.1017/S0140525X19001390},
journal={Behavioral and Brain Sciences},
publisher={Cambridge University Press},
author={Gauthier, Jon and Loula, João and Pollock, Eli and Wilson, Tyler Brooke and Wong, Catherine},
year={2019},
pages={e228}
}
Linking human and artificial neural representations of language.
Jon Gauthier and Roger Levy.
EMNLP 2019.
@InProceedings{gauthier2019linking,
author = {Gauthier, Jon and Levy, Roger P.},
title = {Linking human and artificial neural representations of language},
booktitle = {Proceedings of the 2019 Conference on Empirical Methods in Natural Language Processing},
month = {November},
year = {2019},
address = {Hong Kong},
publisher = {Association for Computational Linguistics},
}
A rational model of syntactic bootstrapping.
Jon Gauthier, Roger Levy, and Joshua B. Tenenbaum.
Cognitive Science 2019.
@InProceedings{gauthier2019rational,
author = {Gauthier, Jon and Levy, Roger and Tenenbaum, Joshua B.},
title = {A rational model of syntactic bootstrapping},
booktitle = {Proceedings of the 41st Annual Meeting of the Cognitive Science Society},
month = {July},
year = {2019},
address = {Montreal, Canada},
}
Does the brain represent words? An evaluation of brain decoding studies of language understanding.
{Jon Gauthier and Anna Ivanova}.
2018 Conference on Cognitive Computational Neuroscience.
@InProceedings{gauthier2018word,
author = {Gauthier, Jon and Ivanova, Anna},
title = {Does the brain represent words? An evaluation of brain decoding studies of language understanding.},
booktitle = {Proceedings of the 2nd Conference on Cognitive Computational Neuroscience},
month = {September},
year = {2018},
address = {Philadelphia, Pennsylvania}
},
Word learning and the acquisition of syntactic–semantic overhypotheses.
Jon Gauthier, Roger Levy, and Joshua B. Tenenbaum.
Cognitive Science 2018.
@InProceedings{gauthier2018word,
author = {Gauthier, Jon and Levy, Roger and Tenenbaum, Joshua B.},
title = {Word learning and the acquisition of syntactic--semantic overhypotheses},
booktitle = {Proceedings of the 40th Annual Meeting of the Cognitive Science Society},
month = {July},
year = {2018},
address = {Madison, Wisconsin},
}
A paradigm for situated and goal-driven language learning.
Jon Gauthier and Igor Mordatch.
NIPS 2016 Machine Intelligence Workshop.
@misc{1610.03585,
Author = {Jon Gauthier and Igor Mordatch},
Title = {A Paradigm for Situated and Goal-Driven Language Learning},
Year = {2016},
Eprint = {arXiv:1610.03585},
}
A fast unified model for parsing and sentence understanding.
{Sam Bowman, Jon Gauthier}, Raghav Gupta, Abhinav Rastogi, Christopher D. Manning, and Christopher Potts.
ACL 2016.
@InProceedings{bowman2016fast,
author = {Bowman, Samuel R. and Gauthier, Jon and Rastogi, Abhinav and Gupta, Raghav and Manning, Christopher D. and Potts, Christopher},
title = {A Fast Unified Model for Parsing and Sentence Understanding},
booktitle = {Proceedings of the 54th Annual Meeting of the Association for Computational Linguistics (Volume 1: Long Papers)},
month = {August},
year = {2016},
address = {Berlin, Germany},
publisher = {Association for Computational Linguistics},
pages = {1466--1477},
url = {http://www.aclweb.org/anthology/P16-1139}
}
Around the web
Currently reading